Collaborative Working: A Case Study
Jun 13, 2024
When you ask the engineers at Shadow Robot what they most love about their work, they talk about the cross-disciplinary challenge and variety of the problems they solve.
Dexterous Manipulation and machine learning is a fast moving, dynamic field. Engineering timelines are long, but machine learning advances are moving at lightning speed and every new development is built on layers of knowledge laid down by organisations across a variety of engineering disciplines.
When solving a very general need, such as manipulating objects in the real world, cross-disciplinary collaboration is the only way to move fast enough to build something useful.
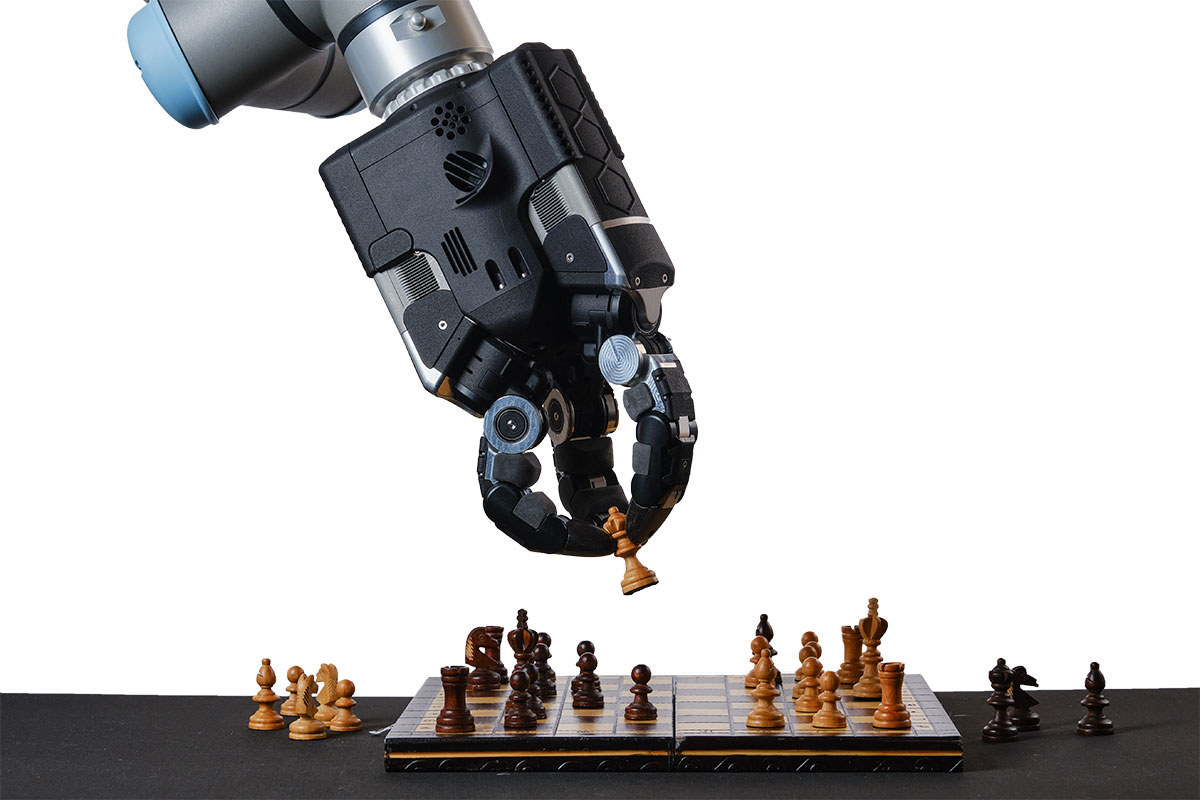
Speccing the new Shadow Hand
Google DeepMind came to Shadow with a very specific request. They were undertaking research into generalised intelligence and the role of embodiment in learning.
Essentially they needed a machine that could repeatedly physically strike its environment in order to learn how to pick things up.
Shadow started working with Google DeepMind in 2017 when they purchased an original Dexterous Hand for their research.
Dexterous Hand’s dexterous manipulation is world-class but it wasn’t built to withstand the multiple physical impacts required as part of this research and Google DeepMind’s learning protocols proved too challenging.
Google DeepMind needed a robot that was reliable enough to run the kind of long-term, physically demanding experiments they need for principled learning. The robot needed to be able to operate in its physical workspace without constraint.
Dexterity was at the core of Google DeepMind’s requirements, but they didn’t specify anthropomorphic hands which gave Shadow lots of opportunity to investigate an optimal solution to achieve their needs around precision, sensing and repairability.
What was required was a sensor-rich machine that could provide the quality and volume of data needed to power their research, and the reliability to ensure that they could run experiments over prolonged periods without losing valuable time to repairs or needing to restart experiments.
An evolving relationship
The project ran over five years, focusing on iterative design and intense testing to find the right solutions.
“When we’re working with researchers, we’re always working at the bleeding edge of the tech,” says Rich Walker. “We’re working with you to support your work; it’s about being responsive and helping clients develop the things they want to do. We don’t just provide a product”.
The project was divided into three phases, each delivering a usable version of the robot for testing before confirming the increasingly complex requirements for the next phase. Starting with a single finger, the team refined every element of the design before developing the final hand.
Delivering a usable robot at the end of each phase was a significant opportunity, not only in terms of engineering, but also in driving the collaborative relationship with Google DeepMind. The team always had a clear understanding of what was needed in the next stage of the project. They could be confident in what had been produced so far and get buy-in for ongoing development. It also meant they had lots of opportunity to adjust the scope, or focus on a specific aspect of the work, so at every stage they could make informed decisions about what to do next.
Rigorous testing
The nature of the project required an aggressive and intensive testing regime. But that process allowed the team to optimise each element of the design before moving on.
By working through feasibility studies and proof of concept for each component, they could move on to the expensive prototyping phase with confidence.
Gavin Cassidy is Shadow’s Head of Product Development. “Working this way means you can test and refine without being constrained by the overall design,” he says. “It’s easier to problem solve like this too, when you can isolate issues one component at a time.
“This was an unusual project because the client was so certain of what they wanted that they could confidently fund the work. It gave us space to push what was required. We could focus on doing things differently, or what we thought would be the main failure points, and really spend time ensuring they were optimal for our needs.”
The tendon system, the kinematics of the hand and the sensor technology all benefited significantly from this approach. In fact Shadow’s optimisation work led Google DeepMind to ask that they dial down the data outputs they were providing.
“When you have an organisation with that kind of resource capability, computation systems and resources asking you to back off a little bit because you’re providing a bit more data than they can use – that’s nice,” says Rich Walker. “It’s nice to feel like we were able to exceed their expectations.”
And the final result is a robot that goes well beyond the functional. “DEX-EE is like no other robot for the work Google DeepMind is doing” says Gavin.
Looking ahead
Shadow’s original Dexterous Hand was developed as a result of Shadow’s own curiosity and desire to see what could be achieved. But DEX-EE is a more intentional product, developed to a client’s requirements with the broader market in mind. Although the confidentiality of the project meant the team couldn’t validate their work with other clients, the deep work they did on development means that the resulting technology will certainly meet the needs of other teams trying to run similar research.
“This feels like the best example of us working on a customer-led project. We engaged with the client to deliver exactly what they needed. Now the work Google DeepMind has done will inspire further research and our technology will be perfectly placed to help,” says Gavin.
And there’s a huge opportunity for non-AI research too.
“The work we’ve done in this project will find its way into future projects across a broad range of contexts. When people see what’s possible we think they’ll see opportunities in industry and applied research where there’s a specific problem to solve and our technology is suddenly the right thing to use.”
Rich is excited to see where the hand takes the Shadow team next.
“For us, the next step is showing this technology to the world and asking, what can you do now that you couldn’t do before?”